Goals-based wealth management: two implementation issues
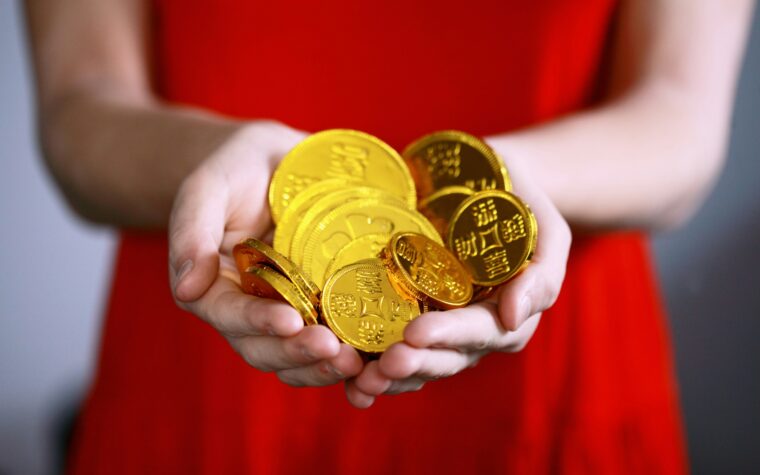
Financial literacy in Europe remains low, influencing individuals’ investment decisions and financial behaviour. To bridge the communication gap between financial advisers and their clients, research has shifted towards a goals-based wealth management approach. Therefore, this paper focuses on the model developed by Das et al. (2022) and addresses two implementation issues when using this model. Firstly, it evaluates the presence of estimation risk. The findings of the perturbation analysis reveal a nuanced picture. However, it can be concluded that errors in the standard deviations of the returns impact the efficiency of the outcomes. Secondly, a six-step process is used to illustrate the different steps when using a goals-based wealth management approach in practice, along with potential pitfalls and mitigation strategies.
1. Introduction
The financial industry is witnessing a global trend towards increased financial responsibility and an increase in retail clients. Despite this, there remains a prevalent lack of financial education among individuals, leading to low levels of financial literacy (OECD, 2017). Behavioural research has indicated that most individuals struggle to comprehend the technical language used by their financial advisers, who often rely on the mean-variance portfolio theory (Das et al., 2018). This highlights a significant communication gap.
To bridge this communication gap between financial advisers and their clients, research has shifted towards a goals-based wealth management approach. Different goals-based wealth management frameworks have been developed. This paper focuses on the one developed by Das et al. (2022), as it considers the different needs of individual investors and uses easy-to-understand language to bridge the communication gap between advisers and their clients. As this algorithm has a broad application area, this paper focuses on two implementation issues of this model.
The first implementation issue this paper addresses is the presence of estimation risk in the goals-based wealth management algorithm of Das et al. (2022). Estimation risk, a well-explored concept in mean-variance theory, evaluates the consequences of using estimated parameters for the necessary inputs, such as expected returns, variances, and covariances. Previous literature has demonstrated that relying on estimates can result in severe over- and underinvestments in asset classes (Chopra & Ziemba, 1993; Chung et al., 2022; Klein & Bawa, 1976). Hence, it is essential to evaluate the impact of this risk within the context of this recent framework. Next, the paper shifts focus to a second implementation issue: enabling the algorithm’s smooth integration into the current state of the financial industry.
2. Goals-Based Wealth Management Methodology
To better understand the goals-based wealth management approach of Das et al. (2022), the model’s inputs and output are explained shortly in this section. The rationale of goals-based wealth management is to use an understandable language to improve the communication between the adviser and the client. Therefore, the inputs of the model used in Das et al. (2022) are understandable to the client and consist of
- Time,
- Pre-determined infusions,
- Initial wealth,
- The efficient frontier accessible to the client,
- The wealth grid, which consists at any point in time of wealth values,
- Cost and utility vectors, which include the costs and utility values, respectively, associated with the goals at a given point in time .
The outputs of the model are the probabilities of attaining the various goals of the investor and the portfolios in which the investor should invest according to its wealth level at a specific point in time. This algorithm uses dynamic programming to maximise the investor’s expected utility by choosing the optimal goals and portfolios.
3. Implementation Issue One: Estimation Risk
In real-world problems, the parameters of return distributions are unknown and should be estimated using historical data (Klein & Bawa, 1976). These estimates are often plugged in as the true parameters, leading to estimation risk. To assess the effect of estimation risk in the market inputs used in the goals-based wealth management model to generate the efficient frontier and the associated investable portfolios, the methodology found in Chopra & Ziemba (1993) and Chung et al. (2022) was used. First, the means, standard deviations and correlation coefficients of the returns were calculated for four different asset classes based on historical data from January 2003 to December 2022. The asset classes used in this paper are the FTSE Euro Broad Investment Grade Corporate Bond Index, the FTSE Europe Government AA Bond Index, the MSCI Emerging Markets Index, and the MSCI European Economic and Monetary Union Index, as they include both bonds and stocks and encompass a wide range of mutually exclusive markets.
Next, errors were included in the three parameters separately. To include errors in the parameters, the methodology of Chopra & Ziemba (1993) was used, which consists of replacing the true mean of asset , , with the approximation where is a standard normal variable and . A larger value for means larger estimation errors. For each value of , 50 iterations with new values of were run. When the mean is changed, the correlation coefficients and the standard deviations are equal to the historical parameters. Next, the correlation coefficients, , and the standard deviations, , were changed similarly. Such perturbation analysis helps identify the parameter that, when affected by an error, has the largest impact on the probabilities of achieving the investor's various goals. This is crucial, as the investor will use these probabilities to decide whether to adjust its input variables or to accept the given probabilities and the associated portfolios.
The initial results are based on the twenty years of available data and an investor of fifty-five years old who wants to use a goals-based wealth management approach until its retirement at the age of sixty-five. The different goals of the investor are represented on a timeline in Figure 1.
Table 1 (see Appendix) provides an overview of the initial results for two cases: when short selling is allowed and when it is not. The table displays the average absolute mean difference between the probabilities of achieving the different goals with and without an error and the average standard deviation of these differences for the different values of , indicating the size of the error, and for the different parameters of the returns that were affected individually (means, standard deviations and correlation coefficients). The table shows that, generally, both the average absolute mean difference and the average standard deviation of the differences increase when the value of increases. Furthermore, the table shows that, in all cases, an error in the standard deviations of the returns leads to larger average absolute mean differences and larger average standard deviations of the differences between the probabilities with and without an error.
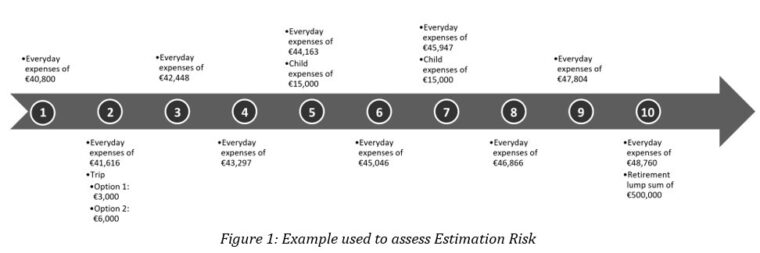
Moreover, it follows from Table 1 that when short selling is allowed and takes on small values, errors in the means lead to smaller average absolute mean differences and smaller average standard deviations of the differences than when errors are included in the correlation coefficients. However, when increases, the reverse is true. Another finding that can be concluded from this table is that an error in the correlation coefficients of the returns does not have a large impact when short selling is not allowed. For the other two parameters, prohibiting short selling leads to larger average absolute mean differences and larger average standard deviations of the differences between the probabilities with and without an error.
Additionally, a robustness analysis consisting of two parts has been conducted. The first part consisted of reducing the time period of available data to the period of January 2008 to December 2022. The second part assessed the impact of changing the goals. The first part failed to support the evidence that the average absolute mean differences are always larger when an error is included in the standard deviations of the returns. The second part of the robustness analysis, on the other hand, supported this evidence. However, in this second part, it was no longer the case that the average values are higher when short selling is not allowed compared to when it is allowed and errors are included in the means or the standard deviations of the returns. Furthermore, it failed to conclude that the average absolute mean difference is larger when an error is included in the correlation coefficients than when an error is included in the means when equals 0.1 and short selling is allowed.
Therefore, the conclusion drawn from this analysis is nuanced. While some evidence suggests that errors in the standard deviations of the returns have a larger effect when short selling is (not) allowed than errors in the means or correlation coefficients, the results are not entirely straightforward. The initial findings indicate that differences between probabilities with an error in the standard deviations of the returns and the probabilities without an error tend to be larger. However, a closer examination through robustness analysis reveals a more complex picture. While the standard deviations of the differences are generally larger in all analyses when errors are included in the standard deviations of the returns, this trend is not consistent across all mean differences. Thus, while there is evidence suggesting a greater effect of errors in standard deviations when short selling is (not) allowed, this conclusion should be approached with caution. When short selling is not allowed, the evidence indicates that errors in correlation coefficients play a minor role, as the differences from the probabilities without an error were consistently small across all analysed cases.
4. Implementation Issue Two: Making Goals-Based Wealth Management Practical
The second implementation issue this paper addresses is how goals-based wealth management can be used in practice. The structure of the industry is changing as digitalisation increases. FinTech and WealthTech make their appearance in the financial industry, and goals-based wealth management can be easily included in these structures. However, some issues remain to be solved when applying goals-based wealth management in practice. Therefore, I structured the goals-based wealth management framework into six steps and looked into different ways to make each step understandable and practical for the client and the adviser. An overview can be found in Figure 2.
The first step consists of identifying the client’s situation. To define its situation, demographic and financial questions should be asked. The initial wealth, as well as the pre-determined infusions, should be known before the goals can be defined, as the goals should be achievable and realistic in the client’s situation. Next, the adviser can help formulate the client’s goals. The SMART principle of Doran (1981) could be used to specify reasonable goals. This principle will also help identify the time horizon and the cost of the goals. In the third step, the desired probabilities of achieving the different goals are defined. This approach helps reduce the communication gap between the adviser and the client. Instead of requiring clients to define the utility associated with each goal—a concept that can be difficult for individual investors to understand—they are asked to specify the probability with which they want to achieve a particular goal.
In the fourth step, the efficient frontier available to the client should be defined together with the wealth grid. For the efficient frontier, it is necessary to specify first the different asset classes in which the investor can invest. These should be in line with the strategy. For the wealth grid, the number of grid points and the bankruptcy wealth need to be determined. These inputs are essential for implementing the goals-based wealth management algorithm described by Das et al. (2022). Next, the algorithm is run, and the results are reported to the client. These obtained probabilities may diverge from the desired probabilities the client has set forward in step three. The client has then different possibilities. Either they accept the lower probabilities, and the algorithm immediately reveals the portfolios the client has to invest in depending on its wealth level at each point in time, or the client changes its desired probabilities, goals, initial wealth, and/or infusions, and the algorithm is rerun. The process is, thus, iterative.
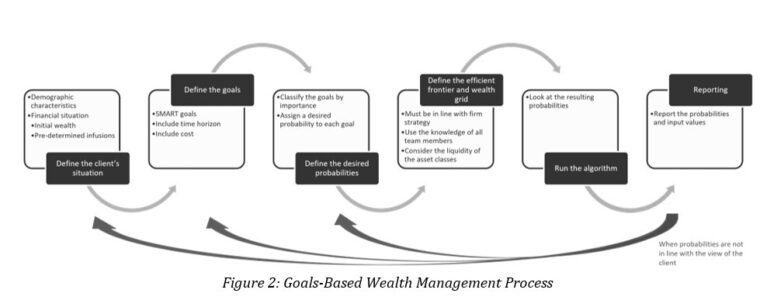
5. Conclusion
This paper addresses two implementation issues when using a goals-based wealth management approach. Such an approach has been developed to reduce the communication gap between financial advisers and their clients.
The first implementation issue this paper addresses is the effect of estimation risk on the probabilities of attaining the various goals of the investor. Such a perturbation analysis has not yet been performed on the multi-goals wealth management algorithm of Das et al. (2022). In this way, this paper contributes to the existing literature. This paper found a nuanced conclusion where the fact that errors in the standard deviations of the returns have a larger effect than errors in the means or the correlation coefficients of the returns cannot be generalised to all scenarios, even though the evidence suggests that errors in the standard deviations have in most cases a larger effect. In most cases, the standard deviations of the differences between the probabilities with an error in the standard deviations of the returns and the probabilities without an error were larger than in the cases with an error in the means or correlation coefficients. However, this conclusion could not be generalised when looking at the mean differences.
The second implementation issue this paper addresses is a way to use goals-based wealth management in practice. A six-step process was developed where each step was discussed using one potential implementation approach. This six-step process has been developed considering the various trends in the financial industry. Consequently, it asserts that integrating a goals-based wealth management algorithm into the digital platforms of financial institutions is viable. Such integration has the potential to offer a competitive advantage, given the industry’s emphasis on personalisation and digitalisation.
Appendix
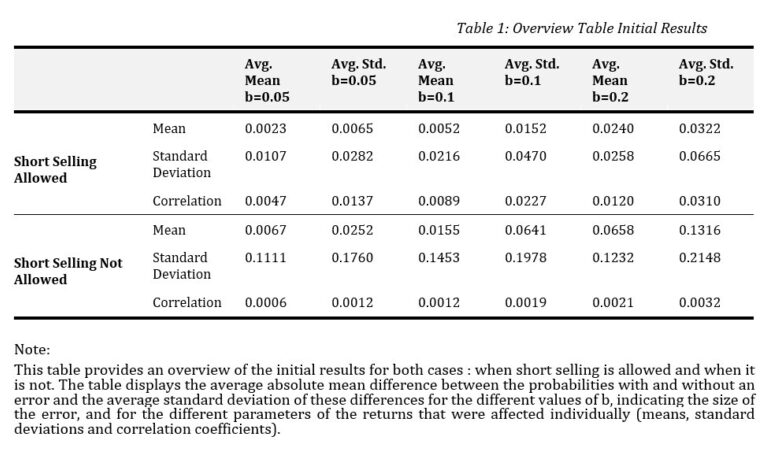
Auteurs
