Determinants of Retail Investor E‑Trading App Usage: Analyzing Economic Factors and the Impact of COVID-19 on E‑Trading App User Numbers (2017−2021)
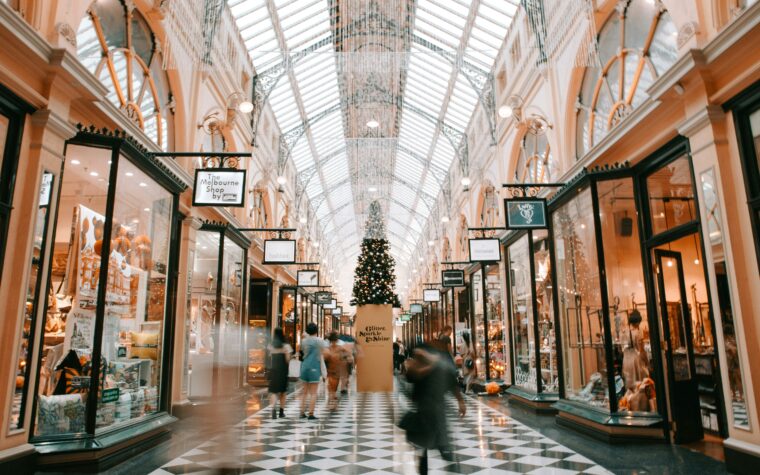
Digital platforms have expanded retail investors’ access to financial markets, making user engagement drivers crucial to understand. Trading apps, facilitating low-threshold access to financial markets, have reshaped this landscape and bolstered retail investor participation. Analysis of a panel dataset from the U.S., U.K., Germany, and France reveals that monthly income positively predicts E-Trading app usage, with higher incomes driving greater retail market involvement. Factors like unemployment rates, mortgage rates, and stock market returns were not statistically significant in predicting app usage. Furthermore, the COVID-19 pandemic also showed a substantial positive impact, underscoring the potential influence of major events on retail investor activity and trading app usage.
Research Background
In today’s digital age, platforms that facilitate access to financial markets for retail investors are becoming increasingly popular.(1) Trading apps, defined as mobile applications that enable the buying and selling of financial instruments such as stocks, bonds, and cryptocurrencies provide users with real-time market data and the tools to execute trades directly from their mobile devices.(2) Various trading platforms offering such services to retail investors make use of zero-commission trading, this entails that these platforms eliminate brokerage fees for buying and selling stocks and other securities, making it more affordable and appealing for individuals to engage in trading activity, which in turn makes investing more accessible to a broader audience.(3)
The widespread adoption of trading apps by retail investors has considerably transformed the financial markets landscape, giving retail investors substantial influence over market dynamics. A retail investor is an individual who engages in trading activities in financial markets for personal investment purposes.(4)The increasing level of retail investor influence over financial markets is exemplified by high-profile events such as the GameStop saga, which dominated headlines for weeks and resulted in questions with regard to the power and influence that retail investors hold over financial markets.(5)
As illustrated in the graph below, which aggregates the number of monthly active users of leading E-Trading apps in the period January 2017 up until June 2021 (54 months) globally, we are able to deduct that the number of retail traders has experienced remarkable growth in recent years, underscoring the (numerical) significance of this emerging investor demographic.(6)
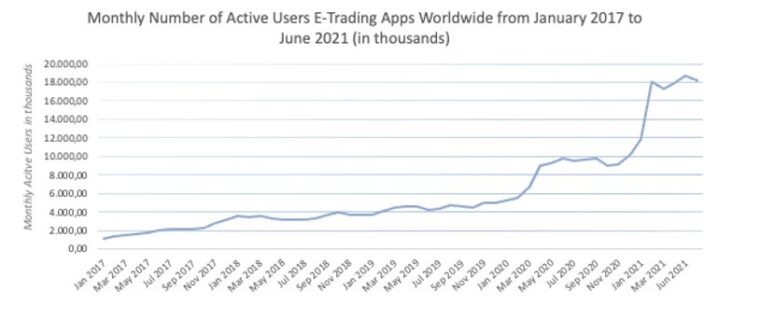
Furthermore, retail investor activity and the (subsequent) usage of trading apps were significantly impacted and affected by the COVID-19 pandemic due to several reasons such as increased market volatility which created opportunities that attracted new investors seeking short-term gains, stay-at-home orders and reduced social activities (giving people more time to engage with online trading platforms), economic uncertainty which pushed individuals to look for alternative income streams, and the widespread shift to digital solutions which highlighted the accessibility and appeal of trading apps during a period when physical services and interactions where less accessible.(7) Furthermore, in the United States stimulus packages further spurred additional stock market investments and participation levels.(8)
Therefore, against this backdrop, our study focuses on the economic (or ‘financial’) situation of consumers (i.e. retail investors) as well as the effect of COVID-19 on retail investor user numbers of leading trading apps. To do so, data on user levels in the period January 2017 up until June 2021 (54 months) was gathered from the following four territories: the United States (US), the United Kingdom (UK), Germany, and France. To be able to single out the determining factors and their effect on the number of users of leading trading apps in the respective territories.
Methodology and Data
Given that this study aimed to analyze the relationship between the number of active monthly users of E-Trading apps and various economic and non-economic indicators, use is made of the panel data model. This model allows to capture both cross-sectional variations and time-series variations, which in the context of this study, provided a more comprehensive understanding of the relationship between the variables, meaning that the data from the four territories was aggregated over the studied time frame, allowing us to obtain more accurate and robust results by leveraging more data points.
Therefore, based on the collected data on user-level numbers, four different models were constructed, one for each studied territory (US, UK, Germany, and France), and data was collected for the aforementioned territories. This resulted in a model consisting of 1728 combined individual data points, facilitating the creation of an accurate and substantiated correlation and regression model.
For this study, the chosen data collection method was to make use of secondary data. This is primarily due to its efficiency and accessibility. Secondary data from reputable sources was utilized, such as government publications and commercial data providers, which enabled the creation of a high-quality dataset with data that has been rigorously collected and verified. Moreover, the usage of secondary data allowed for conducting longitudinal and cross-sectional analyses with a broader scope. Through the use of secondary data, a higher level of comparability and consistency was maintained in our research findings, benefiting from standardized methodologies and well-documented datasets that facilitate more accurate and generalizable results. Additionally, the decision to use secondary data was further validated given the nature of the independent variables, which include the average monthly income, inflation, consumer confidence, interest rates, unemployment rates, and the index returns in the (four) respective territories. These economic indicators are routinely collected by national and international agencies, such as: Statista, Trading Economics, ECB, World Bank Group, OECD, S&P Global, the European Mortgage Federation, and domestic agencies such as the Bank of England, Bureau of Labour Statistics and the Federal Reserve.
The dependent variable of the regression model was the number of monthly active users of E-Trading apps in the studied territories. Furthermore, the dependent variable is transformed into its logarithmic form to facilitate the analysis of percentage changes in the number of monthly active users. The (independent) variables included in the model are:
- Monthly Income, as retail investors with higher incomes tend to trade more frequently, with their financial situation directly affecting their ability to invest.(9)
- Consumer Price Index (CPI), as inflation erodes purchasing power and, when high, increases market volatility, potentially affecting retail trading activity (and app usage).(10)
- Unemployment Rate, as job security and employment levels, influence disposable income and trading activity.(11)
- Consumer Confidence Index (CCI), which measures consumer sentiment and drives market behavior, where higher confidence leads to increased consumption and investment.(12)
- Stock Market Index Returns, as strong stock market performance attracts more retail investors, with positive returns driving greater participation. We used the following indices to construct this variable: S&P 500, DAX, CAC40, and FTSE100.(13)
- Mortgage Rate, where lower rates free up disposable income, encouraging more retail investment through savings from reduced payments.(14)
- COVID-19, a dummy variable indicating either the absence or presence of the COVID-19 pandemic.(15)
The aim of the research questions in the context of this study was to empirically investigate and research the influence that economic and other key factors as well as the COVID-19 pandemic had on the number of (monthly) active users of the leading E-Trading apps in four different territories: the US, Germany, France and the UK. Based on the prior literature review, the following hypotheses were formulated:
(H1) Improving economic conditions (higher income, absence of high inflation, high consumer confidence, etc.) for consumers (i.e. retail investors) leads to higher E-Trading app user levels.
(H2) The COVID-19 pandemic had a significant positive effect on the number of monthly active users of E-Trading apps.
Obtained Results
Concerning the first hypothesis, that improving economic conditions for consumers leads to higher E-Trading app user levels, mixed evidence was found with regard to this hypothesis based on the regression model used. Strong evidence was found to suggest that (average) monthly income and CPI are significant predictors of the number of monthly active users. Additionally, the consumer confidence index was marginally significant, indicating some relationship, though not a very strong one. However, other variables, such as unemployment, mortgage rates, and index returns, did not show statistically significant effects within this model.
Following the second hypothesis, the COVID-19 pandemic had a significant positive effect on the number of monthly active users of E-Trading apps. The pandemic was found to have had the most substantial positive impact on the level of users in the model. To express this in a percentage: the presence of COVID-19 resulted in an increase in the number of monthly active users of 65.9% over the studied period. This is particularly interesting and further confirms previous research which stated that the COVID-19 pandemic, a so-called “black swan event”, and its effects on equity markets show “no significant similarity to any previous event”.(16)
Furthermore, with regard to the observed correlation coefficients, a moderately positive correlation with monthly income suggests that as average income, and thus disposable income, increased, so did the number of users. Similarly, a moderately positive correlation with the COVID-19 pandemic points to heightened activity on trading apps during this period. While positive correlations are observed for mortgage rates, index returns, and unemployment, they are relatively weak, particularly for index returns and unemployment. Conversely, a strong negative correlation with Consumer Confidence Index (CCI) suggests that declines in consumer confidence, likely driven by the pandemic's onset and its economic fallout, spurred a rise in new users on E-Trading platforms, reflecting an increase in investment activity amid economic uncertainty.
These findings highlight the complexity of factors influencing E-Trading app usage and suggest that while certain economic indicators like income and price levels (CPI) are key drivers, other factors may play less of a role than initially expected. The strong impact of the COVID-19 pandemic underscores the importance of external shocks in shaping consumer behavior in financial markets. Overall, this study contributes to a deeper understanding of the multifaceted nature of retail investor activity, emphasizing the need for further research to explore the interplay of economic conditions, market dynamics, and unexpected global events in influencing (digital) trading platform engagement.
However, it is important to acknowledge other factors that also affect retail investor activity, through trading apps, which were not included in our model such as financial literacy, differences in age or income groups, developing countries (rather than the Western countries in our model), the accessibility of trading apps, as well as the fact that our model does not account for trading apps or services offered by domestic (retail) banks.(17)These limitations highlight areas where further research could deepen our understanding of the dynamics at play in the adoption and usage drivers
References
(1) Chong, L., Ong, H., & Tan, S. (2021). Acceptability of mobile stock trading application: A study of young investors in Malaysia. Technology in Society, 64, 101497. https://doi.org/10.1016/j.techsoc.2020.101497; Briere, M. (2023). Retail Investors’ Behavior in the Digital Age: How Digitalization is Impacting Investment Decisions. SSRN. https://doi.org/10.2139/ssrn.4506007; Fan, L. (2021). Mobile investment technology adoption among investors. International Journal of Bank Marketing, 40(1), 50–67. https://doi.org/10.1108/ijbm-11-2020-0551.
(2) Smith, J., & Doe, J. (2020). Mobile Trading Applications: A Review and Analysis. Journal of Financial Technology, 15(3), 120-135. doi:10.1234/jft.2020.15.3.120.
(3) Mihm, S. (2020, January 3). The death of brokerage fees was 50 years in the making. Bloomberg. https://www.bloomberg.com/view/articles/2020-01-03/how-nyse-went-from-quasi-cartel-to-zero-fee-stock-trading?embedded-checkout=true.
(4) Palmer, B. (2023, August 11). Institutional Investors vs. Retail Investors: What’s the Difference? Investopedia. https://www.investopedia.com/ask/answers/06/institutionalinvestor.asp.
(5) Fisch, J. E. (2022). GameStop and the Reemergence of the Retail Investor. European Corporate Governance Institute. https://www.ecgi.global/sites/default/files/working_papers/documents/final_9.pdf.
(6) Statista. (2022, December 12). Monthly number of active users selected leading apps that allow for online share trading worldwide from January 2017 to July 2021, by app. https://www.statista.com/statistics/1259822/global-etrading-app-monthly-active-users/.
(7) Ortmann, R., Pelster, M., & Wengerek, S. T. (2020). COVID-19 and investor behavior. Finance Research Letters, 37, 101717. https://doi.org/10.1016/j.frl.2020.101717; Talwar, M., Talwar, S., Kaur, P., Tripathy, N., & Dhir, A. (2021). Has financial attitude impacted the trading activity of retail investors during the COVID-19 pandemic? Journal of Retailing and Consumer Services, 58, 102341. https://doi.org/10.1016/j.jretconser.2020.102341.
(8) Greenwood, R., Laarits, T., & Wurgler, J. (2022). Stock Market Stimulus. Harvard Business School. https://www.hbs.edu/ris/Publication%20Files/Stock%20Market%20Stimulus_b6da0d1a-2e48-4a6d-a74b-09facf19d2fe.pdf.
(9) Bui, D. G., Hasan, I., Lin, C., & Zhai, R. (2022). Income, trading, and performance: Evidence from retail investors. Journal of Empirical Finance, 66, 176–195. https://doi.org/10.1016/j.jempfin.2022.01.006.
(10) Geske, R., & Roll, R. (1983). The Fiscal and Monetary Linkage between Stock Returns and Inflation. The Journal of Finance, 38(1), 1–33. https://doi.org/10.1111/j.1540-6261.1983.tb03623.x.
(11) Chomicz-Grabowska, A. M., & Orlowski, L. T. (2021). Financial market risk and macroeconomic stability variables: Dynamic interactions and feedback effects. Journal of Economics and Finance, 45(1), 89-109. https://doi.org/10.1007/s12197-020-09505-9.
(12) Mazurek, Jiří & Mielcova, Elena. (2017). Is consumer confidence index a suitable predictor of future economic growth? An evidence from the USA. E+M Ekonomie a management. 20. 30-45. 10.15240/tul/001/2017-2-003.
(13) Schmitz, P., & Weber, M. (2012). Buying and selling behavior of individual investors in Option-Like securities. Social Science Research Network. https://doi.org/10.2139/ssrn.2055088
(14) Andersen, S., D’Astous, P., Martínez-Correa, J., & Shore, S. H. (2022). Responses to Eliminating Saving Commitments: Evidence from Mortgage Run-offs. ideas.repec.org. https://ideas.repec.org/a/wly/jmoncb/v54y2022i5p1369-1405.html.
(15) Ozik, G., Sadka, R., & Shen, S. (2021). Flattening the illiquidity curve: Retail trading during the COVID-19 lockdown. Journal of Financial and Quantitative Analysis, 56(7), 2356–2388. https://doi.org/10.1017/s0022109021000387.
(16) Phadnis, C., Joshi, S., & Sharma, D. (2021). A Study of The Effect of Black Swan Events on Stock Markets – and Developing a Model for Predicting and Responding to them. Australasian Accounting Business and Finance Journal, 15(1), 113–140. https://doi.org/10.14453/aabfj.v15i1.8.
(17) Rooij, M., Lusardi, A., & Alessie, R. (2007). Financial literacy and stock market participation. SSRN Electronic Journal. https://doi.org/10.2139/ssrn.1014994; Khan, M. T. I., Tan, S., & Chong, L. L. (2017). Perception of past portfolio returns, optimism and financial decisions. Review of Behavioral Finance, 9(1), 79–98. https://doi.org/10.1108/rbf-02-2016-0005; Bucher-Koenen, T., Fessler, P., & Silgoner, M. A. (2024). Households’ financial resilience, risk perceptions, and financial literacy – evidence from a survey experiment. SSRN Electronic Journal. https://doi.org/10.2139/ssrn.4726164; Chong, L.-L., Ong, H.-B., & Tan, S.-H. (2021). Acceptability of mobile stock trading application: A study of young investors in Malaysia. Technology in Society, 64. https://doi.org/10.1016/j.techsoc.2020.101497; Rooij, M., Lusardi, A., & Alessie, R. (2007). Financial literacy and stock market participation. SSRN Electronic Journal. https://doi.org/10.2139/ssrn.1014994.
Auteurs

